EE3901/EE5901 Sensor Technologies Week 11 NotesLight sensors and applications to near infrared spectroscopy
We previously studied the photodiode, which uses the photovoltaic effect to generate current in response to light. We also briefly mentioned the light dependent resistor (also called the photoresistor), where the conductivity of a semiconductor is varied by photogenerated charge carriers. In addition to these sensors, there are several other light sensing methods that are important to know about.
Avalanche photodiodes
An avalanche photodiode (APD) is a photodiode designed for low light applications. It is more sensitive than a regular photodiode. As the name suggestions, an APD operates using the avalanche effect. The avalanche effect occurs when electrons are accelerated under a very strong electric field. If the field is strong enough, then the electrons reach a high enough velocity that they can dislodge other electrons upon collisions with atoms in the semiconductor. The simplified picture is that a colliding electron knocks another electron out of the valence band, resulting in the creation of an electron-hole pair. The newly freed electron and hole are quickly separated due to the strong electric field. In essence, this process turns one electron into two electrons (plus a hole). These two electrons are in turn accelerated, and upon collision dislodge yet more electrons. This process is shown in Figure 1. When used for photodetection, the result is that a very high current is generated in response to a small amount of light.
Overview of the avalanche effect, where high-energy electrons dislodge other electrons, such that the current is multiplied.
Zoom:A practical device that utilises the avalanche effect is shown in Figure 2. Its operation is best understood by first considering the layer structure. There is a p-n+ junction known as the multiplication region, which is where charge carriers will be accelerated to high velocity to enable the avalanche effect. This region has a high electric field because it is the depletion region of a p-n junction that has been driven to a very high voltage in reverse bias.
The n+ layer in the multiplication region is more strongly doped than the p layer, so the depletion region extends further into the p region than it does into the n+ region. As the bias voltage increases, the depletion region becomes wider. Eventually, the bias voltage becomes so large that the depletion region reaches the edge of the p-doped layer. Any additional voltage beyond this point will be taken across the intrinsic absorption region. The overall electric field distribution is shown in Figure 2b.
(a) Layer structure of an avalanche photodiode, where “p” and “n” mean p-type and n-type, respectively, “i” means intrinsic (undoped), and the plus sign indicates strongly doped. (b) Approximate electric field distribution within the device relative to the layers drawn above.
Zoom:Avalanche photodiodes operate at very strong reverse bias voltages (e.g. 20 - 90 V depending upon the design). The gain is typically in the range of
The output is a current signal, and so you may be tempted to use a transimpedance amplifier as per a normal photodiode. However, the high bias voltage presents a challenge. A detailed discussion of how to design interface circuits for APDs is given in Linear Technology Application Note 92 by Jim Williams.
Single photon avalanche diodes
It is possible to push the concept of an APD even further, to create an avalanche diode so sensitive that it can be used to detect even a single photon of light. These devices are called single-photon avalanche diodes. The principle of operation is that the device is maintained in an unstable state in reverse bias above the breakdown voltage where it is not yet conducting. This situation is unstable and even a single charge carrier can trigger the avalanche breakdown. Unfortunately, however, the avalanche can also occur spontaneously. Therefore, the detection of a single photon is a probabilistic effect.
The operation of a single photon avalanche diode is best understood via the IV curve in Figure 3. Once breakdown occurs, the current must be quenched by reducing the bias voltage, and then the detector restored back to high bias. Hence there is a recovery time before the detector can be used again.
The current-voltage curve of a single photon avalanche photodiode.
Zoom:The charge carrier that triggers the avalanche can be photogenerated (the desired sensing effect) or thermally generated (an undesired noise signal). Hence single photon detectors have a “dark count rate” meaning the number of times the sensor triggers per second even in complete darkness. Typical values in real devices can be in the kHz range, i.e. the sensor only holds the reverse bias for ~milliseconds before it spontaneously breaks down and has to be quenched and reset.
CMOS imaging sensors
CMOS (complimentary metal-oxide-semiconductor) imaging sensors are found in most modern digital cameras and phone cameras. This type of sensor can be manufactured using the same process as other modern microelectronics, hence CMOS image sensors have benefited from the same technology improvements as other integrated circuits.
Each pixel inside a CMOS sensor consists of a photodiode and multiple transistors that implement buffering and readout circuitry. The simplest design is the three transistor cell, as shown in Figure 4.
Circuit diagram for the “3T” (three transistor) pixel. The capacitor
There are various improvements to this design that use additional transistors, but the essence of operation can be understood using the “3T” (three transistor) cell. The operation of this circuit is as follows.
Firstly, the Reset signal is pulsed high, so that the capacitor
- “Soft reset” where
and the photodiode charges up to where is the transistor threshold voltage. - “Hard reset” where
and the photodiode can be charged to .
Once the capacitor is charged up, the Reset signal is returned to 0, thereby isolating
Meanwhile,
Each individual pixel is read out by pulsing its select pin. In typical use there are “row” and “column” selectors, as per Figure 5.
Schematic overview of how multiple pixels can be combined to create a digital camera.
Zoom:Every pixel in each row has the same “Select” input, and every pixel in each column has the same
To build a colour camera, optical filters are used to select red, green and blue light for specific pixels. The arrangement of red, green and blue pixels varies, but often there are more green pixels because human vision is more sensitive to green light. An example layout is shown in Figure 6.
Example pixel layout for a colour camera.
Zoom:The colour values are interpolated during image processing to produce the illusion of having an RGB channel for every individual pixel.
Light sensing applications: near infrared spectroscopy (NIRS)
Near-infrared spectroscopy is an analytical method based upon the absorption, emission, or reflection of light in the near-infrared region. It can be used to detect the chemical composition of a sample. The near-infrared region is defined by the wavelength range of 800 - 2500 nm. In comparison, the visible spectrum is 380 - 780 nm. Near-infrared light is invisible to the eye but can be measured using photodetectors made from a low-bandgap semiconductor like InGaAs (indium gallium arsenide).
A near infrared spectrometer is an instrument that measures light intensity as a function of wavelength. The basic mechanism of operation is to use a prism or diffraction grating to split the light by wavelength (Figure 7). Each photodetector receives a different wavelength of light, and the instrument can simultaneously record the intensity at multiple wavelengths.
The basic mechanism of a spectrometer, whereby light is split into a spectrum so that the intensity of different wavelengths can be detected.
Zoom:A spectrometer measures a single spectrum, e.g. there are hundreds of photodetectors but they all measure light that came from the same place. With suitable focusing lenses this setup measures the spatial average of the incoming light. A lab bench spectrometer might average
over several
On the other hand, it is often desirable to create an image that maps out spectral properties. This is achieved by an instrument called a hyperspectral camera. The most common implementation (for near infrared spectroscopy) is to use a line scan (Figure 8). A narrow slit of light (representing one spatial axis) is dispersed by wavelength (representing the spectral axis), and directed onto a 2D array of photodetectors.
Different approaches to multispectral imaging. A point scan contains no spatial information, whereas the other mechanisms capture both spatial and spectral data. A line scan images a thin strip of the sample, and the camera or sample must be physically moved in order to build up the image.
(Image created by Lucasbosch and used under the Creative Commons Attribution-Share Alike 4.0 International license.)
The hyperspectral camera records a single row of the image at a time. To record information along the other spatial axis, either the sample or the camera must be moved. In industrial settings this can be achieved by putting the product onto a conveyor belt and passing the moving belt under the camera. There are also drone-mounted hyperspectral cameras where the drone flies at a fixed speed and hence scans the camera past the scene to be imaged.
The output from a hyperspectral camera is sometimes called a “datacube”. It is a 3D array of dimensions height
Interpreting NIR spectroscopy data
Reflectance variation in the NIR region results from energy absorption by organic molecules. There are energy levels associated with vibrations and bending of chemical bonds, which interact in various ways to create variation in the NIR region. Occasionally there are simple interpretations, as shown in Figure 9. In this case, the difference between oily and watery materials is easily determined based on absorption bands of oxygen-hydrogen (O-H) and carbon-hydrogen (C-H) bonds.
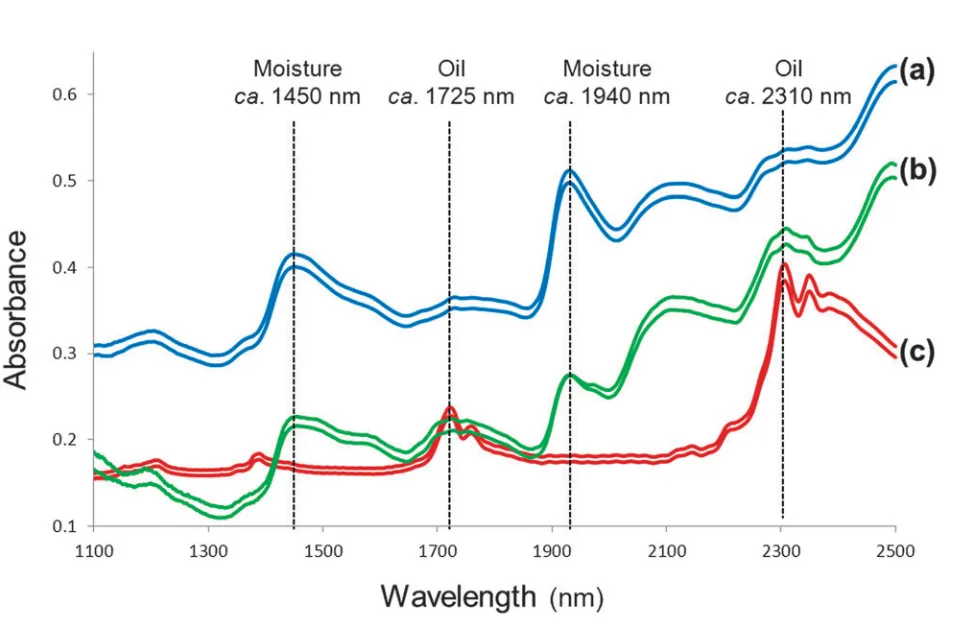
Near-infrared absorption spectra of (a) herbal tea, (b) ground pepper, and (c) olive
oil. The labelled wavelengths correspond to O-H (“moisture”) and C-H (“oil”) chemical bonds.
(Figure by M. Manley, “Near-infrared spectroscopy and hyperspectral imaging: Non-destructive analysis of biological materials,” Chem. Soc. Rev., vol. 43, no. 24, pp. 8200–8214, 2014. Used under a Creative Commons Attribution 3.0 Unported license.)
However, in many practical cases, NIR spectra do not have simple interpretations. Consequently, statistical methods are used to build calibration models. NIR spectroscopy can be considered an indirect method of chemical analysis, since a statistical model must be first trained on results that were measured using an alternative method. However, NIR spectroscopy has strong advantages that it is rapid, does not require contacting the sample, and can measure many things simultaneously by processing the same spectrum through different calibration models in software.
The development of NIR spectroscopy and hyperspectral image analysis is an entire field of research, but as a starting point you can use a simple pipeline consisting of these steps:
-
Process each pixel in the hyperspectral image separately (i.e. treat every pixel as an independent spectrum and neglect position information).
-
Preprocess the spectra by normalising them so each wavelength has a mean of 0 and a standard deviation of 1, i.e. for each wavelength calculate
where
is the mean and is the standard deviation.This method is called “SNV” (standard normal variate). There are other preprocessing steps used in the literature but SNV is the simplest method, and may be suitable for a simple application.
-
Train a statistical model (e.g. regression model). Notice that you need “ground truth” labels obtained by another method, for instance, laboratory analysis.
-
Predict on a new hyperspectral image (that has been preprocessed in the same way, i.e. using the
and from the training set).
A summary of the data flow is shown in Figure 10.
An outline of the data flow for per-pixel hyperspectral image analysis.
Zoom: